Harnessing Data
The adage “you can’t manage what you don’t measure” highlights the crucial role of data in effective decision-making. Earlier articles have emphasized the significance of a structured data infrastructure guided by the DIKW (data, information, knowledge, wisdom) framework. They outlined a comprehensive 10-step process to populate this framework. This article builds on those foundations, focusing on applying these concepts to drive strategic management decisions and enhance customer profitability.
Peter Drucker, a renowned figure in management theory, famously stated that businesses exist to “create a customer.” Drucker’s insight, shared in his Harvard Business Review article “The Information Executives Truly Need,” underscores the necessity for predictive and strategic models. While historical operational data is valuable, executives require forward-looking information to make informed decisions about customer acquisition, retention, and overall profitability.
Businesses need a well-defined approach to measuring customer profitability to effectively manage customer relationships and optimize profitability.
The following is a detailed guide on how to achieve this.
Foundation information
Accurate customer profitability measurement begins with a solid data foundation. Companies should maintain a centralized repository of data that captures both historical and predictive insights related to customer interactions, costs, and revenues. This involves ensuring the data infrastructure aligns with the DIKW framework, which helps transform raw data into actionable insights.
Key metrics for measuring customer profitability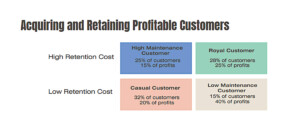
- Net present value (NPV) for acquisition. NPV is a critical metric for evaluating customer acquisition decisions. It involves discounting future cash flows from a customer to their present value, which helps determine the long-term benefits of acquiring that customer or segment. This metric is particularly useful for assessing whether the initial investment in acquiring a customer will yield positive returns over their lifetime. NPV is calculated on a fully loaded basis, considering all potential costs, both fixed and variable, and revenues associated with the customer.
- Net income for existing customers. For existing customer relationships, where historical data is more relevant than lifetime projections, net income is the preferred metric. Net income reflects the excess of revenues over costs during a specific period (monthly, quarterly, or annually). It provides a snapshot of profitability without needing long-term projections, making it ideal for day-to-day decision-making. However, if significant capital expenditures are involved, NPV might be reconsidered for such specific scenarios.
- Customer-centric metrics. Beyond NPV and net income, several other metrics can offer valuable insights into customer profitability:
- Customer lifetime value (CLV): CLV estimates the total revenue a business can expect from a customer over their entire relationship. It helps in understanding the long-term value of retaining a customer.
- Customer acquisition cost (CAC): CAC measures the cost associated with acquiring a new customer. By comparing CAC to CLV, businesses can gauge the efficiency of their acquisition strategies.
- Customer retention rate: This metric tracks the percentage of customers retained over a given period. High retention rates often correlate with higher profitability and lower acquisition costs.
- Continuous monitoring and analysis. Regular monitoring of customer profitability metrics is essential for maintaining strategic alignment. This involves tracking changes in customer behavior, market conditions, and cost structures to refine strategies as needed. Continuous analysis ensures that business decisions remain relevant and effective.
- Data governance and security. Effective data governance is integral to managing customer profitability. Proper governance ensures that data is organized, accurate, and accessible only to authorized individuals. It also involves complying with internal and external regulations regarding data privacy and security. Data governance frameworks help businesses maintain the integrity of their customer data and make informed decisions based on reliable information.
The role of AI in management decision-making
Artificial intelligence (AI) is increasingly becoming a pivotal tool in everyday life and management decision-making. Films like “2001: A Space Odyssey” have explored the impact of AI on human decision-making as far back as 1968, often depicting both its potential and its risks. While AI has the power to analyze vast amounts of data and offer predictive insights, businesses must ensure that AI algorithms are well-defined, thoroughly audited, and transparent. Confidence in AI-driven decisions hinges on understanding and trusting the underlying algorithms and data used.
In conclusion, managing customer profitability effectively requires a combination of strategic foresight, accurate data, and continuous analysis. By implementing the right metrics and maintaining a solid data governance policy, businesses can make informed decisions that attract and retain valuable customers and optimize overall profitability. As AI continues to evolve, it will play an increasingly significant role in these processes, making it essential for businesses to approach AI with both enthusiasm and caution.
For now, focusing on solid data practices and strategic metrics will provide a strong foundation for enhancing customer profitability and driving sustainable business growth. As we look towards the future, we will discuss leveraging AI in everyday decision-making.
Jon Hill is the CEO of Cobotiq and provides business managers with information on how to create and implement profitability. He is a frequent speaker and presenter on the future impact of automation and technology in the cleaning industry.